The Power of Image Labeling in Business Growth
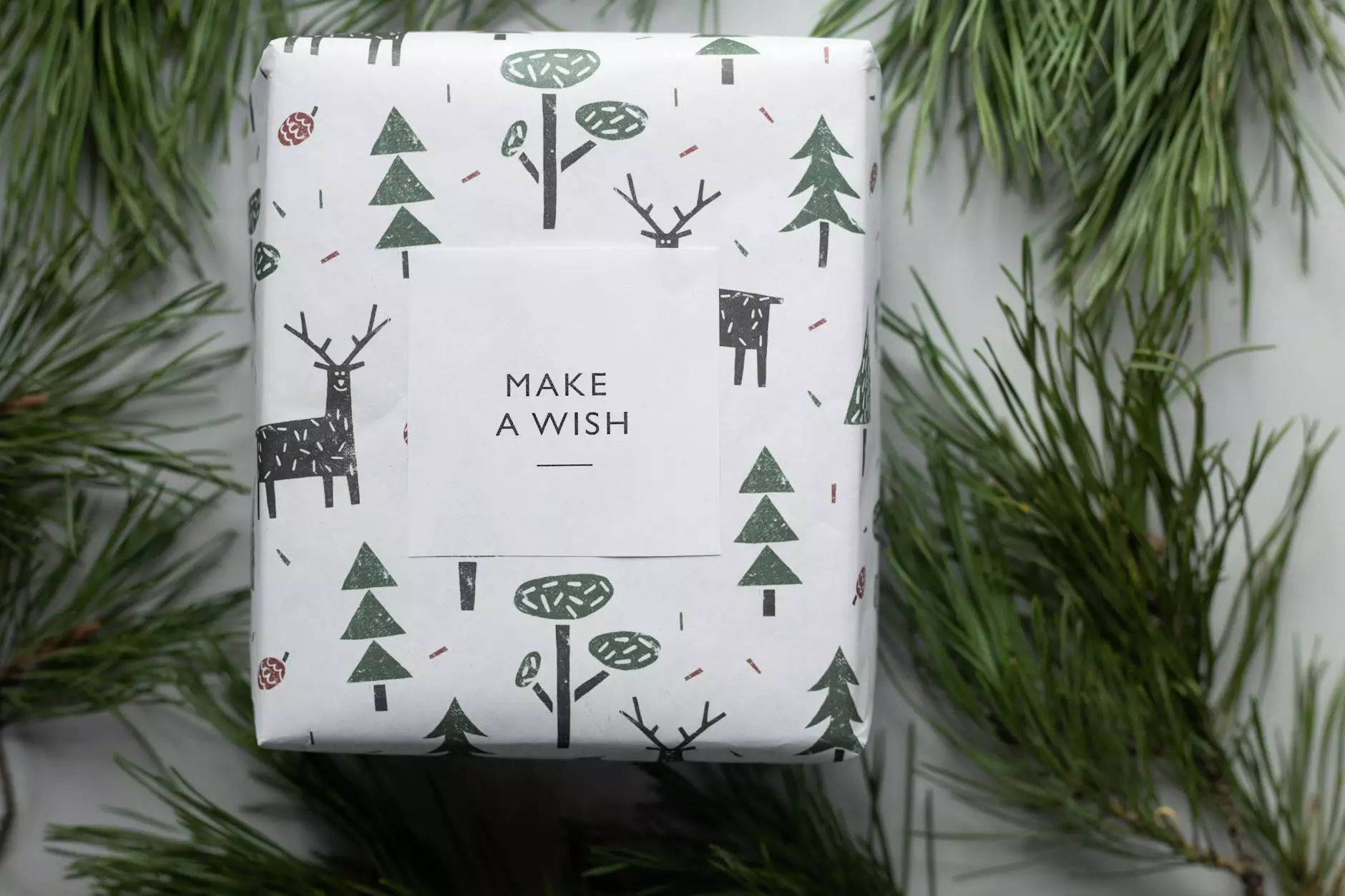
In today's fast-paced digital landscape, businesses are constantly seeking innovative ways to harness the power of technology to improve operational efficiency and drive growth. One of the most vital technologies emerging at the forefront of this transformation is image labeling. This article explores the critical role of image labeling in software development, the techniques involved, and how it can propel businesses towards success.
Understanding Image Labeling
Image labeling is the process of annotating images to identify objects, actions, and other attributes within those images. This practice is essential for training machine learning models, especially in the field of computer vision. Machine learning algorithms require large datasets to learn and make accurate predictions. Image labeling provides these datasets, enabling models to understand and interpret visual information.
The Importance of Image Labeling in AI
As artificial intelligence (AI) continues to evolve, the demand for accurate and high-quality image data has surged. Here are several reasons why image labeling is crucial in AI development:
- Enhanced Accuracy: Properly labeled images improve the accuracy of AI models, allowing them to make better predictions and decisions.
- Data Diversity: A diverse set of labeled images helps algorithms generalize well to new, unseen data, preventing overfitting.
- Improved Detection: Accurate image labeling is essential for applications such as facial recognition, autonomous vehicles, and medical imaging, where precision is paramount.
- Scalability: Businesses can scale their AI projects effectively by integrating labeled datasets, which leads to broader applications of technology.
How Image Labeling Works
The process of image labeling can be broken down into several steps, each crucial to ensuring high-quality outputs. Let's examine each of these steps in detail:
1. Data Collection
The first step is gathering a wide range of images. The quality and diversity of these images determine the effectiveness of the model. Businesses should aim to collect images from various sources and formats to create a comprehensive dataset.
2. Annotation Guidelines
Before labeling, it's essential to establish clear annotation guidelines. These guidelines ensure that the labeling process is consistent and meets the project’s objectives. Explicit criteria help to avoid discrepancies among annotators and improve the quality of the labeled data.
3. Labeling Process
The actual labeling can be done manually or through automated tools. Each method has its pros and cons:
- Manual Labeling: This process involves human annotators who review and label images. It offers high accuracy but can be time-consuming.
- Automated Labeling: Leveraging AI tools can speed up the labeling process significantly. However, the initial accuracy may depend on the sophistication of the tool and its training data.
4. Quality Assurance
To maintain high-quality datasets, it's essential to implement quality assurance processes. This may involve random sampling of labeled images to check for accuracy and consistency. Feedback loops can also help improve annotator performance.
5. Integration into Models
Once the images are labeled and quality-checked, they can be integrated into the machine learning models. This integration allows the models to learn from the labeled data and enhances their performance in real-world applications.
Benefits of Image Labeling for Businesses
Investing in image labeling provides numerous benefits for businesses across various sectors. Here are some compelling advantages:
- Enhanced Decision-Making: With better-trained AI models, businesses can make more informed decisions based on accurate data analysis.
- Increased Efficiency: Automated systems powered by well-labeled images streamline operations, reducing manual labor and associated costs.
- Competitive Edge: Organizations that leverage advanced image recognition technologies can gain a significant advantage over competitors who do not.
- Better Customer Experience: Businesses can utilize image labeling in applications like recommendation systems to provide personalized experiences for customers.
Applications of Image Labeling in Various Industries
The applications of image labeling are vast and impactful across various industries. Here’s how different sectors utilize this technology:
1. Retail and E-commerce
In retail, image labeling aids in inventory management and visual search capabilities. By labeling product images, retailers enable customers to search visually for items, improving user experience and boosting sales.
2. Healthcare
In the healthcare industry, accurate image labeling is crucial for medical imaging, helping to identify anomalies in X-rays, MRIs, and other scans. By training AI models with well-labeled medical images, healthcare professionals can improve diagnostic accuracy.
3. Automotive
Autonomous vehicles rely heavily on image labeling for object detection and recognition. Labeled images help train models that enable vehicles to identify and react to pedestrians, traffic signs, and other cars, enhancing safety on the roads.
4. Agriculture
In agriculture, image labeling is applied in precision farming. Farmers utilize labeled drone imagery to assess crop health, monitor growth, and detect pests or diseases early, leading to higher yields and better resource management.
Choosing the Right Image Labeling Solution
Finding the right image labeling solution is paramount for businesses looking to integrate this technology. Here are some considerations to keep in mind:
- Quality of Labeling: Ensure that the service or software provides high-quality labeling that meets your specific needs.
- Scalability: The solution should be able to scale with your business, accommodating growing data needs effectively.
- Customization: Look for solutions that allow for customizable labeling frameworks to align with your business requirements.
- Size of the Team: For manual labeling, the size and expertise of the annotator team can impact the quality and speed of the output.
The Future of Image Labeling in Software Development
As we move forward, image labeling will continue to evolve alongside advancements in technology. Here are a few future trends to anticipate:
1. Increased Automation
With advancements in AI and machine learning, we can expect more automated labeling solutions that will dramatically reduce the time and cost associated with the labeling process, leading to quicker turnaround times for businesses.
2. Improved Tools
Emerging tools are likely to provide more intuitive interfaces for annotators and incorporate AI-driven suggestion features, enhancing labeling efficiency and accuracy.
3. Broader Applications
As more industries recognize the benefits of image labeling, its applications will broaden to include new technologies such as augmented reality (AR) and virtual reality (VR), providing fresh avenues for innovation.
4. Heightened Data Privacy Considerations
In an era where data privacy is paramount, businesses will need to implement stringent measures to protect labeled datasets, ensuring compliance with regulations such as GDPR while leveraging the benefits of image labeling.
Conclusion
In conclusion, image labeling stands as a cornerstone in software development and AI applications, providing businesses with the essential data needed to train robust models. As companies like Keymakr continue to refine their approaches to image labeling, the potential for enhanced accuracy and efficiency in operations will only grow. By investing in quality image labeling solutions, businesses can unlock new opportunities for innovation, improving their competitive edge and ensuring long-term success in the digital economy.